Pyramid's game-changing NLQ Chat Bot lets users analyze data in whole new way
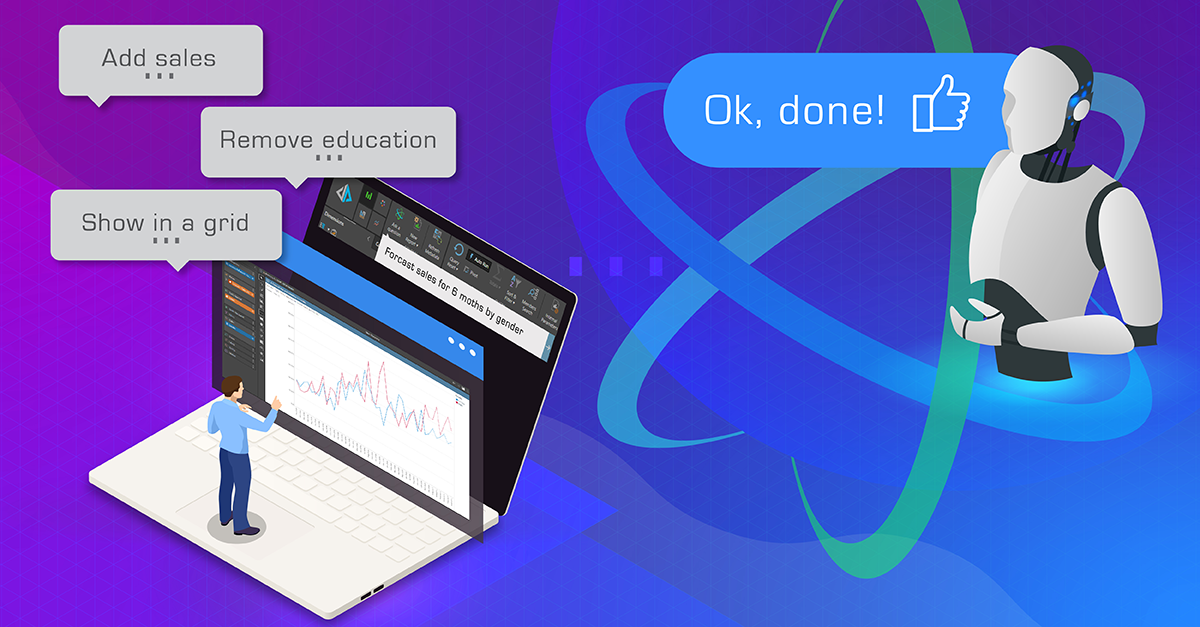
Chat bots are everywhere these days. While commonly associated with customer experience use cases, their application extends beyond answering basic customer questions. In the BI world specifically, they assist non-technical users by responding with analytical results in the form of visualizations, without requiring them to click buttons or perform the drag-and-drop functions common in these applications.
The primary challenge with the BI chat bot use case is that analytical data is idiosyncratic—it’s very specific to each business and dataset. Further, if chat bots are to become ubiquitous in BI workflows, their analytic capabilities and performance need to be portable to all of an organizations’ different database technologies. In other words, it cannot only support specific (or proprietary) datasets.
Pyramid Analytics has long-offered natural language query (NLQ) capabilities. The NLQ Chat Bot in our 2020.13 release allows any type of user to interact with existing visualizations and analytics—running on any supported database.
The problem
Many non-technical users are uncomfortable using even basic point-and-click and drag-and-drop BI actions, because even basic skills require training. Most are also unfamiliar with more complex—but still common—BI features like drill, dice, swap, filter, and sort, although they intuitively recognize the need to use them in analytics scenarios. Further, most users are unaware of data structures, hierarchies, and measures, which often limits their ability to use natural language queries to build data visualizations on their own.
To solve this, many BI solutions have added natural language querying that is flexible enough to compensate for most users’ limited knowledge of BI, as well as the underlying data characteristics. However, most BI tools’ natural language offerings only work on proprietary technologies and are unable to render the feature in direct query mode on other databases. They also tend to lack the depth of functionality to handle more advanced analytical questions.
What’s more, they often lack true “language skills”—relying too much on premeditated “prompting.” They only provide basic answers to basic questions—displaying over-simplistic visualizations that don’t provide any real insights, nor allow users to make further changes to visualizations, either through direct modification to the data visualizations or additional queries.
Pyramid’s solution
Pyramid’s NLQ Chat Bot works directly on any supported data source (SQL, MDX, et cetera) and is not constrained to a proprietary data engine or the underlying data size or data structure. The Chat Bot‘s intuitive and flexible plain English approach to report-building lets everyday users build data visualizations without requiring traditional analytics and BI actions.
By “chatting,” users can interact with and interrogate data with common functions like drill, dice, swap, filter, and sort. In addition, Pyramid’s NLQ Chat Bot offers convenient prompts to users derived from key elements in the data model. This “augmented” assistance lets them ask the right questions without having to know the underlying data structures, hierarchies, and measures.
In addition, once the Chat Bot has produced an initial visualization, non-technical users can evolve the data views by gradually adjusting previous questions in the session. Queries can be reset to their original state or re-built from scratch. Plus, with Pyramid’s NLQ Chat Bot, users can insert wildcards and keywords to dynamically adjust.
The Chat Bot uses a neural network dependency parser to group words and phrases together, determining nouns and verbs, without relying on a prompting model. It includes abilities to handle word derivatives (“stemming”) and has a built-in thesaurus to better find alternatives to words found in real-world scenarios.
Business case
Diana, the CFO for D&R Retailers, is using Pyramid to perform analytics on her Redshift data warehouse. She would like to make several ad-hoc queries independently without asking BI analysts to assist her. However, she is not tech savvy or familiar with the nomenclature and structure of the underlying data models she regularly accesses.
D&R have been running marketing campaigns aimed at professional married couples and she wants to see how effective they have been. Diana starts an analysis using the Discover NLQ search bar using the “ask a question” button and writes her first query: “show sales by occupation and marital status.”
Pyramid automatically responds by producing a graph and organizes the chips in the correct drop zones.
Pyramid determines the best visual and instantly produces the result.
Next, Diana wants to compare the sales to expenses for all products using a scatter plot. She again poses the question in simple terms: “compare sales to total expenses in a scatter plot by product by year, promotion category.”
Using the NLQ AI, Pyramid finds the optimal way to produce the result, arranging the chips accordingly and drawing the result.
Diana would now like to forecast what sales amounts are expected for the next six months by gender. She writes: “forecast sales for 6 months by gender.”
And again, Pyramid presents a clear visual using its built-in forecasting algorithms.
After reviewing her dashboards, Diana focuses on her “Occupation” visual that analyzes returns and net profit per occupation. She expands it to full screen for further analysis.
From here, Diana activates the NLQ Chat Bot again to gain further insight. Unlike the standard NLQ, the chat bot accumulates changes to an existing report. So Diana decides to review cost figures by simply typing in “add cost”—which adds cost data to the existing report.
Next, Diana wants to view the most active promotions, so she simply types “show top 5 promotions.” The result takes the previous query, bolts on the top five promotions, and redraws the grid.
Next, she wants to see a year-on-year comparison, so she adds “compare this year to last year.” The NLQ engine understands that its 2020, so it auto-creates two logical periods for 2020 and 2019 and appends it to the analysis.
Diana now wants to only view the information for New York, California, and Texas. Without needing to specify the columns in the data model, Pyramid recognizes the city names and finds the geographical data matching the request to generate the appropriate query.
Next, Diana wants to simplify her analysis and chooses to remove cost.
She also decides to swap returns data with sales data. Pyramid uses its in-built thesaurus to find “Revenue,” since “sales” doesn’t exist.
Diana uses the prompt intellisense to select promotion data as she adds months and removes the promotion column.
To view a product breakdown and to further compress her report, Diana removes the State and Occupation columns and adds the Product column. Even though she used the term “merchandise” instead of “product,” the thesaurus resolves the selections.
And now Diana decides to only focus on 2020 figures, so she cuts the clutter and instructs via a chat note.
She further simplifies her report by removing gender and product.
Diana wants to change the grid to a chart visual so she tells the bot to “flip to a line chart.”
Despite being an analytics novice, Diana’s simple questioning has produced analytic content as if she was a pro.
Summary
Chat bot usage has become widely adopted in answering “knowledge” type questions. In analytics, chat bots can assist non-technical users to build and display visualizations without requiring technical abilities or explicit knowledge of data structures, hierarchies, and measures. Today, many BI tools’ natural language offerings work on proprietary data engines only, relying on “prompting” rather than “language” with simplistic capabilities at best.
Pyramid’s NLQ Chat Bot works directly on any supported data source regardless of the size or structure of the data, using plain English, with advanced language handling and analytic functions.
This post originally appeared at https://www.pyramidanalytics.com/blog/details/blog-pyramid-s-game-changing-nlq-chat-bot-lets-users-analyze-data-in-whole-new-way
Other Resources
Natural Language Querying (NLQ) [Video]
NLQ Chat Bot [Video]
1 reply
-
We like the new chat bot because it works on every database we have without having to duplicate the data. We tried out thought-spot, only to realize that we need to copy the entire data warehouse into their data engine before our users could even try it out - which ended up costing a fortune in our AWS deployment.
Content aside
- 2 Likes
- 4 yrs agoLast active
- 1Replies
- 131Views
- 2 Following